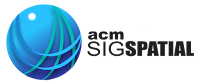
Building upon our previous work on Location-Based Social Networks (LBSNs) and how agent-based modeling could provide an alternative to real world data sets, in the latest SIGSPATIAL Special Newsletter, we (Joon-Seok Kim, Hamdi Kavak, Chris Rouly, Hyunjee Jin, Dieter Pfoser, Carola Wenk, Andreas Zufle and myself) have an article entitled "Location-Based Social Simulation for Prescriptive Analytics of Disease Spread."
In this article we discuss a geographically explicit agent-based model that we have been developing that is capable not only of simulating human behavior but also able to create synthetic but realistic LBSN data based on human patterns-of-life. Furthermore, in the article we discuss how such data and models can be used to explore the parameter space of possible prescriptions to find optimal strategies (or policies) to achieve a desired system state and outcome. We refer to such a search for optimal policies as prescriptive analytics. (for readers wishing to learn more about prescriptive analytics please see the 1st ACM KDD Workshop on Prescriptive Analytics for the Physical World).
To give an example of such prescriptions, in the article we make use of a simple hypothetical disease model and explore two prescribed policies to mitigate the spread of the disease. The first policy requires all agents to wear simulated Personal Protective Equipment (PPE) that reduce the chance of infection by 50%. The second policy enforces strict social distancing measures onto a fixed proportion of 50% of the population. Those who follow the social distancing order avoid recreational site visits from meeting people although they still go to restaurants. In addition to these two policies, as a baseline, we also ran a “null-prescription” in which no intervention was prescribed. We find that the social distancing prescription was extremely effective. On the other hand, our simulation results for PPE policy showed that merely wearing protective gear without any change in behavior has no significant effect (for the case of this disease).
If this type of research is of interest to you, below we provide the abstract to the paper, a movie of a representative simulation run, some of our results of the prescriptions described above and a link to the paper itself. Further information about the model and data can be found at https://geosocial.joonseok.org/p/epidemic.html and the data is available at https://osf.io/e24th/. Also as we are currently going through COVID-19, we thought a a brief write up and links to some disease models and discussions of modeling efforts related to it was also appropriate to include.
In this article we discuss a geographically explicit agent-based model that we have been developing that is capable not only of simulating human behavior but also able to create synthetic but realistic LBSN data based on human patterns-of-life. Furthermore, in the article we discuss how such data and models can be used to explore the parameter space of possible prescriptions to find optimal strategies (or policies) to achieve a desired system state and outcome. We refer to such a search for optimal policies as prescriptive analytics. (for readers wishing to learn more about prescriptive analytics please see the 1st ACM KDD Workshop on Prescriptive Analytics for the Physical World).
To give an example of such prescriptions, in the article we make use of a simple hypothetical disease model and explore two prescribed policies to mitigate the spread of the disease. The first policy requires all agents to wear simulated Personal Protective Equipment (PPE) that reduce the chance of infection by 50%. The second policy enforces strict social distancing measures onto a fixed proportion of 50% of the population. Those who follow the social distancing order avoid recreational site visits from meeting people although they still go to restaurants. In addition to these two policies, as a baseline, we also ran a “null-prescription” in which no intervention was prescribed. We find that the social distancing prescription was extremely effective. On the other hand, our simulation results for PPE policy showed that merely wearing protective gear without any change in behavior has no significant effect (for the case of this disease).
If this type of research is of interest to you, below we provide the abstract to the paper, a movie of a representative simulation run, some of our results of the prescriptions described above and a link to the paper itself. Further information about the model and data can be found at https://geosocial.joonseok.org/p/epidemic.html and the data is available at https://osf.io/e24th/. Also as we are currently going through COVID-19, we thought a a brief write up and links to some disease models and discussions of modeling efforts related to it was also appropriate to include.
Abstract:
Human mobility and social networks have received considerable attention from researchers in recent years. What has been sorely missing is a comprehensive data set that not only addresses geometric movement patterns derived from trajectories, but also provides social networks and causal links as to why movement happens in the first place. To some extent, this challenge is addressed by studying location-based social networks (LBSNs). However, the scope of real-world LBSN data sets is constrained by privacy concerns, a lack of authoritative ground-truth, their sparsity, and small size. To overcome these issues we have infused a novel geographically explicit agent-based simulation framework to simulate human behavior and to create synthetic but realistic LBSN data based on human patterns-of-life (i.e., a geo-social simulation). Such data not only captures the location of users over time, but also their motivation, and interactions via temporal social networks. We have open sourced our framework and released a set of large data sets for the SIGSPATIAL community. In order to showcase the versatility of our simulation framework, we added disease a model that simulates an outbreak and allows us to test different policy measures such as implementing mandatory mask use and various social distancing measures. The produced data sets are massive and allow us to capture 100% of the (simulated) population over time without any data uncertainty, privacy-related concerns, or incompleteness. It allows researchers to see the (simulated) world through the lens of an omniscient entity having perfect data.