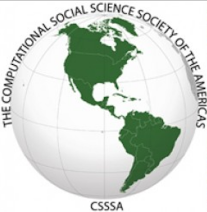
Continuing our work on machine learning and agent-based modeling, at the upcoming Computational Social Science (CSS 2020) annual conference, Dale Brearcliffe and myself have a paper entitled: "Creating Intelligent Agents: Combining Agent-Based Modeling with Machine Learning." In the paper we discuss how advances in computational availability and power have permitted a rapid increase in the development and use of machine learning (ML) solutions in a wide variety of applications (some examples we have already shown on this website), including within agent-based models.
One thing however, is that while within the ML community at large, it is common to compare different approaches and take the one that gives the best result (e.g., like we did in the Communities, Bots and Vaccinations paper), this is not the case within the social simulation community. There has been little written with respect to why one ML method was chosen over another, or how the simulation results might be different if different ML methods were used. To address this gap we demonstrate the integration of three machine learning methods (i.e., Evolutionary Computing, Q Learning, and State→Action→Reward→State→Action (SARSA)) into the well-known agent based model: Sugarscape (in this instance we modified NetLogo's "Sugarscape 2 Constant Growback"). Our rationale for choosing the Sugarscape model was that it is well known within the social sciences and as the purpose of this paper was not to solve or explore a specific social issue, but to show how different ML methods can be used within the same agent-based model and to show how different methods impact the results of a model.
If this type of research is of interest, below we provide the abstract to the paper, a flow chart of the model execution along with some results. At the bottom of the past you can find the full reference and a link to the paper. Supplementary material can also be found at https://tinyurl.com/ML-Agents. At this link, the model presented in this paper along with a full description of it following the Overview, Design concepts, and Details (ODD) protocol can be found. We do this to allow others to replicate the results and adapt the ML methods for their own applications if they so desire.
One thing however, is that while within the ML community at large, it is common to compare different approaches and take the one that gives the best result (e.g., like we did in the Communities, Bots and Vaccinations paper), this is not the case within the social simulation community. There has been little written with respect to why one ML method was chosen over another, or how the simulation results might be different if different ML methods were used. To address this gap we demonstrate the integration of three machine learning methods (i.e., Evolutionary Computing, Q Learning, and State→Action→Reward→State→Action (SARSA)) into the well-known agent based model: Sugarscape (in this instance we modified NetLogo's "Sugarscape 2 Constant Growback"). Our rationale for choosing the Sugarscape model was that it is well known within the social sciences and as the purpose of this paper was not to solve or explore a specific social issue, but to show how different ML methods can be used within the same agent-based model and to show how different methods impact the results of a model.
If this type of research is of interest, below we provide the abstract to the paper, a flow chart of the model execution along with some results. At the bottom of the past you can find the full reference and a link to the paper. Supplementary material can also be found at https://tinyurl.com/ML-Agents. At this link, the model presented in this paper along with a full description of it following the Overview, Design concepts, and Details (ODD) protocol can be found. We do this to allow others to replicate the results and adapt the ML methods for their own applications if they so desire.
Abstract.
Over the last two decades with advances in computational availability and power, we have seen a rapid increase in the development and use of Machine Learning (ML) solutions applied to a wide range of applications, including their use within agent-based models. However, little attention has been given to how different ML methods alter the simulation results. Within this paper, we discuss how ML methods have been utilized within agent-based models and explore how different methods affect the results. We do this by extending the Sugarscape model to include three ML methods (evolutionary computing, and two reinforcement learning algorithms (i.e., Q Learning, and State→Action→Reward→State→Action (SARSA)). We pit these ML methods against each other and the normal functioning of the rule-based method (Rule M) in pairwise combat. Our results demonstrate ML methods can be integrated into agent-based models, that learning does not always mean better results, and that agent attributes considered important to the modeler might not be to the agent. Our paper's contribution to the field of agent-based modeling is not only to show how previous researchers have used ML but also to directly compare and contrast how different ML methods used in the same model impact the simulation outcome, which is rarely discussed thus, helping bring awareness to researchers who are considering using intelligent agents to improve their models.
Keywords: Agent-based Modeling, Evolutionary Computing, Machine Learning, Reinforcement Learning, Sugarscape.
Full reference:
Brearcliffe, D.K. and Crooks, A.T. (2020), Creating Intelligent Agents: Combining Agent-Based Modeling with Machine Learning, The 2020 Computational Social Science Society of Americas Conference, Online. (pdf)
أكثر...